Day Zero Ethics for Military AI
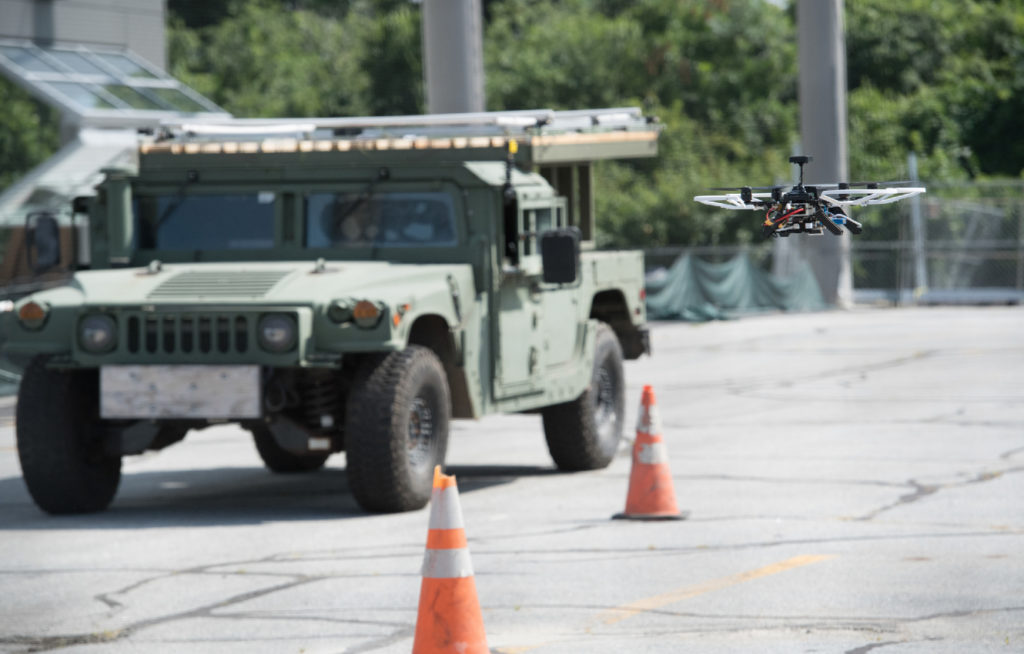
Editor’s Note: This article was submitted in response to the call for ideas issued by the co-chairs of the National Security Commission on Artificial Intelligence, Eric Schmidt and Robert Work. It addresses the third question (parts b. and d.) which asks authors to consider the ethical dimensions of AI.
Examining the legal, moral, and ethical implications of military artificial intelligence (AI) poses a chicken-and-egg problem: Experts and analysts have a general sense of the risks involved, but the broad and constantly evolving nature of the technology provides insufficient technical details to mitigate them all in advance. Employing AI in the battlespace could create numerous ethical dilemmas that we must begin to guard against today, but in many cases the technology has not advanced sufficiently to present concrete, solvable problems.
To this end, 2019 was a bumper year for general military AI ethics. The Defense Innovation Board released its ethical military AI principles; the National Security Commission on AI weighed in with its interim report; the European Commission developed guidelines for trustworthy AI; and the French Armed Forces produced a white paper grappling with a national ethical approach. General principles like these usefully frame the problem, but it is technically difficult to operationalize “reliability” or “equitability,” and assessing specific systems can present ambiguity — especially near the end of development.
Given the wide-ranging potential applications and challenges presented by AI, the Department of Defense and its contractors should tackle legal and ethical concerns early and often in the development lifecycle, from the formative stages of an AI concept to its realization and eventual deployment. Only by considering legal and ethical principles long before Acquisition Milestone A will AI capabilities reflect enduring American principles. Ethical considerations should shape future system requirements, and developers should recognize both technical and ethical challenges. Incorporating ethics analysis could reshape development processes and create new costs for developers, but decision-makers and developers alike must recognize that an “early and often” approach has tangible benefits, including aiding in future compliance reviews like Defense Department Directive 3000.09.
The early and often principle developed by moving legal and ethical discussions from the ivory tower to the battlefield. Our team at the Institute for Defense Analyses is tackling this challenge as part of the Defense Advanced Research Projects Agency’s (DARPA) development of the Urban Reconnaissance through Supervised Autonomy program. This is not a weapons system: It is intended to move ahead of a patrol, using AI and autonomy to discern potential threats and sources of harm to U.S. forces and civilians. A multidisciplinary research group of academics, philosophers, lawyers, military experts, and analysts has incorporated law and ethics to analyze the system’s technological dependencies and components from its inception. This analytical process could be applied to other systems and offers one path forward for ethical military AI development.
Shaping System Requirements
Holistically considering the legal, moral, and ethical implications of future AI-enabled and autonomous systems early and often first requires bridging a conceptual gap. Assessments must break down the possible and plausible, examining both a system’s ideal performance in operation and its real ability to perform a task. Analyzing ethical strengths and weaknesses requires the assessor to understand a system’s purpose, its technical components and their limitations, relevant legal and ethical frameworks, and the system’s efficacy at a task compared to that of a human operator.
In reality, assessing ethical compliance from design to deployment resembles a spiral model, requiring repeated testing, prototyping, and reviewing for technological and ethical limitations. The viability of any AI system ultimately will be assessed when it is employed. Choices implemented early in the system’s design — such as dependence on neural nets for image recognition of human behavior — carry legal and ethical implications for the system’s reliability in the field, particularly when things go wrong.
Legal and ethical considerations require broadening requirements from the purely technical (e.g., computer vision, image recognition, decision logic, and vehicle autonomy) to include international humanitarian law, the laws of war, and relevant tort rulings. For example, international humanitarian law requires discriminating between combatants and civilians, and dictates that unknown individuals be considered civilians. To comply with the law, an AI-enabled system that is uncertain of an individual’s status would need to check with a human operator before acting in a way that might cause disproportional harm to that individual. This alone requires developers at the outset of a system’s design to analyze human-machine agency trade-offs, account for decision-to-action latency times, and incorporate into technical designs sufficient time for operators to review machine decisions. Ultimately, the mutual reinforcement of ethical and technical requirements drives developers’ plans by enshrining the principle that design and development must be informed by an understanding of ethical issues that could arise in the field.
As forward-looking legal and ethical considerations shape requirements across the board, developers will find it necessary to consult experts or even multidisciplinary teams throughout the design and development process. In addition to pointing out legal red lines and flagging areas of ethical concern, these experts could help develop other key features of ethical analysis. Two such key elements are system explainability and transparent documentation.
Emphasizing System Explainability and Ethical Documentation
DARPA’s Heilmeier Catechism is a collection of thought exercises to help agency officials dissect proposed programs with straightforward questions. For example, without using jargon, what is your system trying to do? What are the limits of current practice? What are the risks involved?
These questions are at the heart of what could be defined as a system’s “explainability.” In this case, we are not referring to explainability in a forensic sense of understanding the underpinnings of deep-learning systems. Rather, at the outset of system development, developers should also be able to describe how their system will function in the field, including the objectives it aims to achieve and the tasks it will undertake, the technologies it will rely on to do so, and the technical, legal, and ethical risks inherent to using those technologies. As updates to system designs occur and recur, legal and ethical implications should continuously be reexamined and evaluated. In complex systems of systems, developers’ focus on cracking individual technical components can overshadow considerations of system end-use goals and operational context, thereby leaving these difficult explanatory questions unanswered.
Ethical documentation requirements — essentially requiring a paper trail devoted solely to legal, moral, and ethical concerns — present a simple method for capturing system explainability. Developers should document their systems without jargon and should include critical dependencies, possible points of failure, and gaps in research to ensure that non-technical audiences understand the legal and ethical risks and benefits of new AI-enabled systems. In keeping with the early and often principle, developers will have to consider concepts of operations — how their system will be used in the field — earlier in the design process than is currently typical in order to accurately document their systems. A detailed mission walkthrough (with the aid of tools like process maps) could help developers identify agency hand-off points, system decision points, or design choices for user interfaces and other components that incur potential for bias. Developers are already required to produce risk burn-down documentation to identify and mitigate technical issues for new systems. Similar documentation for ethical risks will ensure that developers are transparently contemplating ethical challenges early in the design process.
Law and ethics-specific documentation would also emphasize the importance of consistent terminology within developer teams throughout the development process. Complex AI-enabled and autonomous systems, which often contain multiple components developed by subcontractors, can confuse people trying to assess the ethical impact of a system, particularly when developers use inconsistent names and definitions for the same components. Assessments that incorporate multidisciplinary teams of civil experts and military specialists can both bridge terminology gaps and highlight areas of potential confusion.
Tackling Research Gaps and Bias
Early and often ethical analysis can also identify gaps in relevant research and point out the potential for system bias while systems are still in development. By identifying research gaps where it would help developers make ethical design choices, decision-makers can allocate resources to studies that address immediate needs. For example, there is a known lack of research on the reliability of AI-enabled image recognition for certain types of human behaviors. As ethical analyses uncover research gaps that might apply across future platforms, upfront research costs could benefit future systems with similar technical dependencies.
Describing the operating environments in which an AI-enabled system will operate often depends on anecdotal recollections of combat experiences. This can serve as a useful starting point for training these systems, but it has limitations. AI is only as good as the data it is trained on. Many machine-learning techniques crucially depend on access to extensive and well-curated data sets. In most instances, data sets incorporating the subtleties and nuances of specific operating environments and conditions simply do not exist. Even where they do exist, they often require substantial effort to convert to formats amenable to machine-learning processes. Further, the AI community has learned the hard way that even well-developed data sets may harbor unforeseen biases that can color the machine learning in ways that raise serious ethical concerns.
Regular ethical analyses can help to address bias issues in the design and development of AI-dependent systems. Such analysis can also serve as a backstop against introducing unintentional bias, whether it occurs via system outputs that bias human operators or via operator bias, into the system’s decision-making processes. Law and ethics assessors can help think through data sets, algorithmic weighting, system outputs, and human inputs to try to identify bias throughout the design process and to serve as sounding boards for developers and subject matter experts alike.
Conclusion
The future of warfare is headed toward autonomy. America and its allies are not the only actors who have a say in what that future looks like. Near peers are using AI in troubling ways, and the importance of trying to establish the rules of the road and abiding by them is paramount to maintaining the unique soft power advantage that the United States and its allies enjoy through adhering to moral and ethical considerations. Laying out these principles and transparently applying them to relevant U.S. military systems will help in establishing best practices within the defense community, developing a common lexicon with allies and partners, and building trust among concerned publics and the tech community. In the end, this will occur not simply as a byproduct of intellectual clarity on legal and ethical issues but as an outgrowth of early and often ethical engagement during system development.
At first glance, applied legal, moral, and ethical considerations seem onerous, particularly where new requirements for personnel or documentation are likely necessary. They may also require the development, acquisition, and operational communities to reevaluate the applicability of their standard operating procedures. However, early and often ethical analysis, comprising continual testing, prototyping, and reviewing areas of legal or ethical concern, will mitigate the rise of ethical considerations that would detrimentally impact later development and acquisition stages and that could prevent system deployment. Facilitating this analysis through improved transparency in system design and improving the explainability of AI and autonomous decision processes will be key to realizing these benefits, particularly as the Department of Defense moves to practical implementation of Directive 3000.09.
Human warfighters learn lessons of ethics, morality, and law within society before they enlist. These lessons are bolstered and expanded through reinforcement of warrior and service-specific ethos. As the U.S. military increasingly incorporates AI and autonomy into the battlespace and we ask intelligent machines to take on responsibilities akin to those of our service personnel, why should we approach them any differently?
Owen Daniels is a research associate in the Joint Advanced Warfighting Division at the Institute for Defense Analyses working on the IDA Legal, Moral, Ethical (LME) AI & Autonomy research effort.
Brian Williams is a research staff member in the Joint Advanced Warfighting Division at the Institute for Defense Analyses and task leader of the IDA Legal, Moral, Ethical (LME) AI & Autonomy research effort.
This research was developed with funding from the Defense Advanced Research Projects Agency.
The views, opinions, and findings expressed in this paper should not be construed as representing the official position of the Institute for Defense Analyses, the Department of Defense, or the U.S. government.
Image: U.S. Air Force (Photo by Todd Maki)