Our Future Lies in Making AI Robust and Verifiable
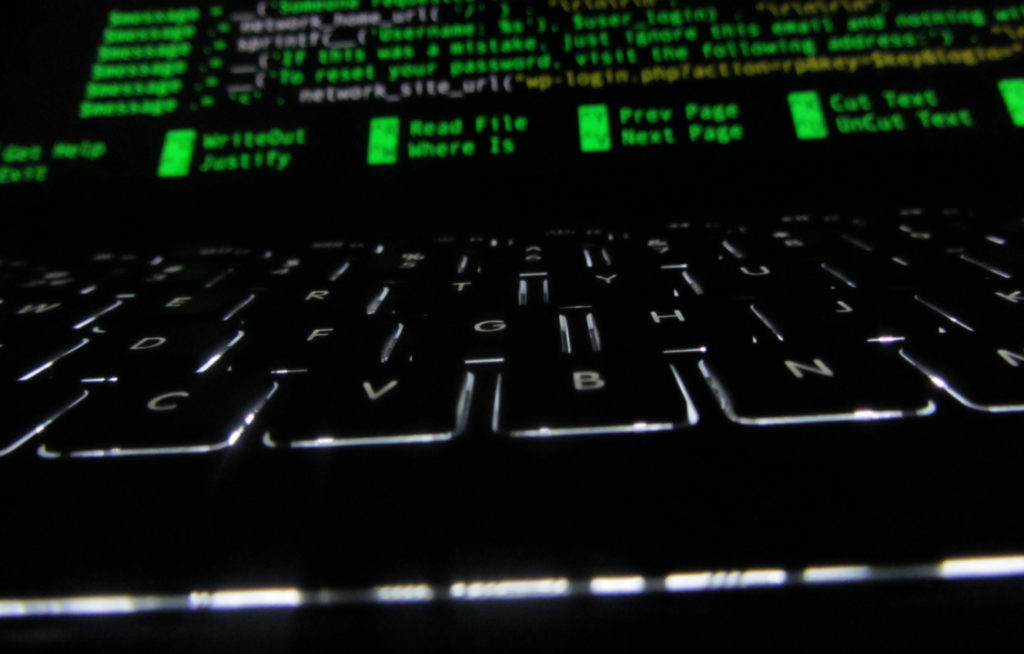
This article was submitted in response to the call for ideas issued by the co-chairs of the National Security Commission on Artificial Intelligence, Eric Schmidt and Robert Work. It addresses the first question (part b.), which asks what might happen if the United States fails to develop robust AI capabilities that address national security issues. It also responds to question five (part d.), which asks what measures should the government take to ensure AI systems for national security are trusted.
We are hurtling towards a future in which AI is omnipresent — Siris will turn our iPhones into personal assistants and Alexas will automate our homes and provide companionship to our elderly. Digital ad engines will feed our deepest retail dreams, and drones will deliver them to us in record time. In the longer term, autonomous cars will zip us around our smart cities where the traffic is fluid and where every resource, from parking spaces to energy and water, is optimized. Algorithms will manage our airspace, critical infrastructure, healthcare, and financial systems. Some technologies promise to detect illnesses earlier and others to develop drugs faster and cheaper. Still other algorithms will be dedicated to protecting our nation and our way of life.
This AI-enabled future is blinding in its possibilities for prosperity, security, and well-being. Yet, it is also crippling in its fragility and can easily come to a screeching halt. All it might take is for a safety-critical AI system to fail spectacularly in the public eye — an AI analog to the Three Mile Island accident, or worse, a series of cascading incidents leading to mass casualties (e.g., AI-enabled traffic lights that malfunction and set in motion a mass-pileup of autonomous vehicles at a busy intersection) — to halt the advancement and adoption of these technologies, and public support for it, in its tracks.
How likely is a scenario of doom and gloom to come to fore? Unfortunately, quite likely, unless the global community takes critical steps to fortify its current approaches for technology development. Algorithms are fragile, and the very science of verification to certify that they perform as desired is still inadequate, especially where black box AI algorithms are concerned. We should not trust what is not robust, and we cannot trust what we cannot verify. The government should take the lead in setting and meeting the standards and expectations for the productive development of AI-enabled safety-critical applications
AI Black Boxes
In a (trained) AI system as a black box that processes inputs and produces outputs, the box is robust if small changes in the input it is fed do not lead to large changes in its output. Robustness is a desirable property of engineered systems — this is usually accepted as a given — and yet designers and users are seemingly forgetting it amidst the whiz-bang enthusiasm for all things AI.
There are “adversarial examples” that fool classifier algorithms with image classification instances that capture the public’s imagination. One example includes a tweaked image of a turtle that is easily recognizable to the human eye but misclassified as a rifle by the algorithm. In another, a modified stop sign, readily recognizable to the human eye, is misclassified as a speed limit sign by the algorithm.
These examples aren’t evidence of particularly clever “adversarial” intent. Indeed, many adversarial examples have been shown to be generalizable across models. That is, the same example will fool many different neural networks. Additionally, black box model attacks against machine learning algorithms, requiring no upfront knowledge of their internal workings, have been demonstrated. These adversarial examples are symptoms of the brittleness and fragility of the algorithms —in other words, their lack of robustness. Moreover, this lack of robustness appears to be pervasive. Indeed, far from being limited to image classifiers, adversarial examples have also been demonstrated for neural network-based text and malware classifiers, among others.
When Safety-Critical AI-enabled Systems Lack Robustness
The lack of robustness in individual algorithms has critical, sometimes deadly, implications when such algorithms are used as part of a wider safety-critical system operating in the physical world.
The utility and power of machine learning advances are compounded when used in an integrated system in the physical world. For example, an image classifier is one of many systems that senses and processes signals in an autonomous vehicle, providing inputs to various feedback loops that control the behavior of this vehicle, from how it plans and follows its path to how it avoids collisions or communicates with other vehicles to optimize traffic flow. What happens to your car when its image classifier mis-reads the stop sign? Maybe the intersection will be empty. But car buyers, and society, will expect more than “maybe.”
Past experience has taught us that when physics and algorithms start interacting, interesting, complex phenomena emerge, hence the continuing research interest in cyber-physical systems. And when physics meets algorithms that lack robustness, the results can be deadly. Consider the X-15 experimental aircraft that splintered in midair minutes after its launch in 1967, killing the pilot. That crash was partly due to a faulty adaptive flight control system. Adaptive control, a nascent field at the time of the accident, is an example of a true “learning” system, one that updates its parameters in real-time and in response to what it senses in the surrounding environment.
Building robustness into systems necessitates, among many other things, a solid grasp of the fundamentals of how these algorithms work and why they fail when they do, one that was sorely missing for adaptive control algorithms in 1967, and one that is sorely missing in the design of today’s machine learning algorithms. Simply put, the science behind the algorithms needs to catch up with their engineering.
The Role and Challenges of Verification
Better design, with attention to robustness, only gets us part way there. Technical checks and balances to certify that the AI-enabled system behaves as desired gets us across the finish line. The science of verification — how we know whether a system will do what we think it will do — remains limited. Even where fundamentals exist, they are unable to grapple with the ever-increasing complexity and scale of the systems being designed and put forth in the world. Indeed, verification of control systems (model-based AI) is an active area of research where complex dynamics, involving non-linearities or discontinuities, are present. Moreover, even when the tools exist, they do not scale well with model dimension. Verification of machine learning systems (data driven, black box AI), particularly deep learning systems, is completely unchartered territory. And when model-based and data-driven algorithms start interacting in safety-critical systems, as they undoubtedly will in many applications (autonomous vehicles for example), the complexity of the verification challenges are multiplied many times over.
Verification may consist of purely mathematical approaches to prove desirable properties, or it may consist of extensive testing or simulation with some underpinning mathematical fundamentals to ensure the system behaves as desirable and expected under reasonable assumptions about operating conditions. While robustness is a property engineers bake into system design, verification is the process or suite of processes that allow us to certify the system that’s been designed. It serves as the technical checks and balances that are run on a system prior to its deployment.
The explosion of the Ariane 5 rocket on its maiden flight and the recent Boeing 737 MAX accidents highlight what happens when verification fails in safety-critical systems employing AI algorithms. Boeing appears to have relied on an algorithm, the Maneuvering Characteristics Augmentation System (MCAS), to address dynamic instability that kicks in at high angles of attack. Moreover, the MCAS appears to have been designed to respond to sensor input from one of the two angle-of-attack sensors on the plane while ignoring all other input — a poor design that does not bode well should that sensor fail.
Verifying safety-critical systems requires going beyond the software implementation of the algorithm. Experts must also get under the hood to verify the algorithms themselves. Indeed, the Ariane example illustrates a verification gap in the software implementation, while that of the Boeing 737 MAX illustrates a verification gap in the algorithms.
Maintaining America’s Competitive Advantage and Our Value Systems
The world is changing, and will be transformed by the possibilities of AI. It is in America’s national interest, and to many across the globe who look to the United States for leadership in this domain, to ensure that it is setting, and meeting, the standards and expectations for the productive development of this technology for safety-critical applications, and for the good of all.
So, how does the United States go about setting and meeting these expectations? And whose responsibility is it? The good news is there are promising starting points, both in classical robust control and within the context of systems with interacting analog (physics) and discrete (computer) components. There are also encouraging developments within the machine learning community and the budding interest in understanding the interaction of machine learning, feedback loops and physics.
But much more can be done. Cooperation and cross-fertilization should occur between research communities that are culturally distinct (e.g., machine learning, control theory, robust optimization, and information theory). This will be invaluable for progress at speed. Vigorous interest in verification and validation, including development of the theory as well as design of experiments and testbeds will be critical. This interest can be, and should be, cultivated and enhanced through well-placed investments in basic and applied research in AI verification and validation as well as infrastructure for testing and evaluation of AI-enabled systems.
While the federal government is responsible for national defense and public safety, the private sector will deploy many of these AI technologies first, and at scale. The scope of the end-goal, the immensity of the challenges ahead, and the risks assumed collectively as a society if and when these systems fail (if these failure are not prevented in advance) demand a whole-of-nation approach.
The Department of Defense, as a major user of safety-critical AI-enabled systems, could spearhead the effort in close partnership with industry and academia. Everyone wins by working together on this. And by doing so, the United States could continue to lead the advancement of this technology globally, in line with its interests and values.
Danielle C. Tarraf, PhD, is a senior information scientist at the nonprofit, nonpartisan RAND Corporation, where her work focuses on technology strategy, informed by quantitative and data-driven analyses. She began her career as an electrical and computer engineering faculty member at Johns Hopkins University, where she established and directed a research lab focused on advancing control theory, particularly as it interfaces with theoretical computer science and reinforcement learning.
CORRECTION: Due to an error by the managing editor, a previous version of this article states, “Unfortunately, quite likely, unless the United States takes critical steps to fortify its current approaches for technology development. U.S. algorithms are fragile. …” This was a mistake, as the author wanted to avoid focusing exclusively on the United States, and instead highlight the global nature of this issue. This has been corrected, and the article now reads, “Unfortunately, quite likely, unless the global community takes critical steps to fortify its current approaches for technology development. Algorithms are fragile. …”