Building Modern Screw-Sloops? Strategic Choices about Artificial Intelligence in Defense
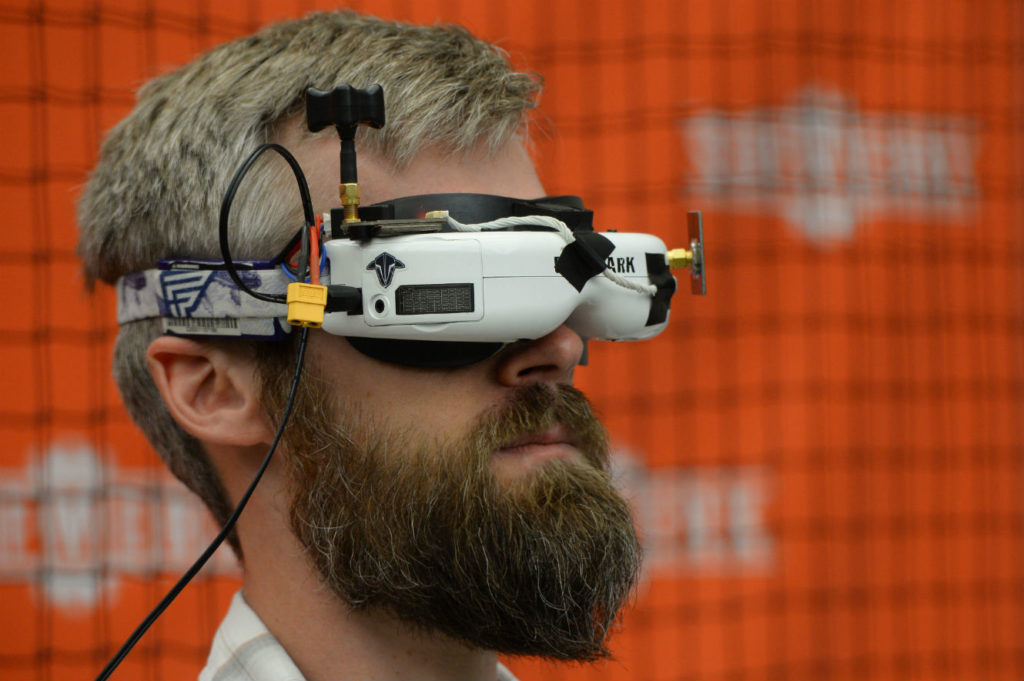
When militaries avoid making choices about new technologies, they often wind up with something like the “screw-sloop”: a hybrid solution that fails to take full advantage of either the old or the new technology. In the mid-1800s, many navies commissioned screw-sloops, which were warships with both steam propulsion and masts and rigging for sails. One of the best-known screw-sloops, the H.M.S. Warrior, was designed to be the most powerful ship of her day. Commissioned in 1861, she was obsolete a decade later. She languished for over a century as a schoolhouse and later as a floating oil hulk before being converted to a museum ship in the 1980s. Most screw-sloops served about 10 years of useful life as warships before being turned over to administrative duties.
Military technological innovation can be decisive in war, but only if militaries are decisive in peacetime. Militaries are usually conservative outside periods of open conflict. Although they pay lip service to innovation, they prefer to put off hard choices about technological change. We see the same in the hype associated with artificial intelligence (AI). At least for the H.M.S. Warrior, the Royal Navy knew what it wanted to “steamify.” For AI, the U.S. Department of Defense remains stymied at step one: What, specifically does it want to “intelligentize” in warfare? A successful department-wide AI implementation at scale could be more transformative than steam propulsion since its impacts are likely to be more broadly felt. But is the department poised to exploit the potential of AI, or is it on a path to building the equivalent of screw-sloops? The answer depends on the military’s overall risk tolerance and decisiveness in making bold choices right now.
The unclassified summary of the Defense Department’s AI strategy, released on Feb. 12, is an attempt to send a strong public signal: We are on board to try everything and anything that gives us an edge in the emerging great power AI technological race. Like many glossy unclassified defense “strategy” documents, this is a “yes and…” document of non-decision-making. The document itself doesn’t illuminate the difficult training, resource, and political decisions that come with implementation.
The Defense Department has an AI strategy (or at least a vision), which is good. Moving toward achieving that vision requires several additional hard steps, including identifying the gap between the data such a strategy would require and what’s actually available; recognizing that some processes that seem ripe for automation are labor-intensive for a reason; and managing the cultural and practical obstacles to public-private partnerships. Ultimately, the strategy fails to convey how risk-tolerant leaders will be in their efforts to “intelligentize” the department.
Defense resources are a zero-sum game. What are the combatant commands, the Joint Chiefs, and the Pentagon’s senior civilian leadership willing to give up in exchange for the benefits of AI? Here are just a few of the challenging conversations the department needs to have and choices it needs to make:
Data: The Lifeblood of Artificial Intelligences
An effective Defense Department AI strategy requires an effective Defense Department data strategy. Data is the element that feeds the algorithm to make it smart. So what do we want (or already have) to feed our algorithms?
The strategy frames the initial efforts of the recently established Joint Artificial Intelligence Center narrowly within the boundaries of defense systems to be used in key missions — in other words, automating defense. The document envisions “improving situational awareness and decision-making, increasing the safety of operating equipment, implementing predictive maintenance and supply, and streamlining business processes.” Here, the data source seems to be the Pentagon’s internal stores of information. The idea seems to be to create more efficient processes, from manpower to training to logistics, by using all the information stored about Defense Department systems.
But the document later seems to imply that this internal information would be offered up for sharing outside Defense Department systems, calling for open AI missions with academia and industry. These data pools would be “shared” data. Presumably, this means the Defense Department will have to extract, standardize, and, in many cases, digitize the billions of records currently siloed in each of its many offices. Then it has to render those records usable for the private sector. Once that’s done, how does the data flow?
If the data flow goes only one way – from the Pentagon on out – the task is Herculean but not impossible. Bureaucratic politics within the department are likely to result in different and incompatible formats and systems, much like we saw in the inability of the Department of Veterans Affairs and the Department of Defense to agree on a standard for electronic medical records. And once data on service members has been shared, it will be difficult for private companies to resist using it for other commercial purposes. Imagine the potential financial value of health care information that the Defense Department collects to anticipate, prioritize, and respond to threats, or data on the entertainment and purchasing preferences of the active duty force collected to help focus recruiting efforts.
If the data flow goes two ways between the department and the private sector (as the Pentagon will certainly want it to), the challenge becomes much more than bureaucratic. U.S. government and military data strategies are rightly handicapped by limitations on collecting data about U.S. citizens, and government privacy regulations are less efficient and effective than they should be. But a strategy that involves the two-way sharing of data between the Defense Department and private companies like Amazon, Google, or Facebook poses challenging questions of privacy, civil liberties, and civil-military relations: Can the Pentagon purchase data from commercial sources that it would be prohibited from collecting itself? What are the legal and privacy constraints of scrubbing and aggregating metadata to feed AI learning algorithms, and can such data be sanitized and shared?
This is challenging even in a non-combat mission, such as humanitarian assistance and disaster relief . Among the controversial data requirements associated with applying AI to this mission would be public health data to anticipate medical needs, cell phone data to reveal where and how people travel, and crime and business data to anticipate civil disorder. And, given the worry about “losing the AI race,” it is unrealistic to think the Pentagon’s appetite for data will be limited to non-combat missions.
Some of these concerns might be mitigated with data collected from non-U.S. citizens abroad, which intelligence agencies already routinely do, but this raises new concerns about classification and data-sharing, potential “spoofing” of U.S. data collection by adversaries, and the risk that U.S. data collection tools may be missing important parts of the data stream. Addressing issues like these will require advocacy at the national level by the secretary of defense, who must be an informed and effective advocate for Defense Department AI data needs as an issue of grand strategy. To be clear, we are not suggesting that democracies open the floodgates of data surveillance floodgates because of global AI competition. Instead, we are pointing to the real and necessary obstacles to getting the necessary data given that the United States is a democracy and different from China in terms of what information its military can collect.
The Inefficiency IS the System
The department’s AI strategy also seeks to “streamline business process” by reducing the time spent on “highly manual, repetitive and frequent tasks.” One might wonder if the authors of those words have spent much time in the Defense Department, since these goals are at odds with its culture. Stifling and inefficient bureaucratic processes aren’t always accidental vestiges. They often exist because they are useful in the perpetual power struggles between services and for expanding power on either side of the civil-military divide inside the Pentagon.
Some highly manual, repetitive, and frequent business tasks are great candidates for AI, as anyone who has tried to book train travel in through the Defense Travel System would readily agree (readers attempting to click this link will note that their browser will resist with a privacy error due to an outdated certificate; we rest our case). But these clunky processes are as often a feature as a bug. Government contracting and acquisition rules designed to guard against profiteering, graft, and corruption rigidly specify performance metrics and technical details in a way that prevents rapid integration of new technologies. Interface between government software and private databases is similarly regulated by strict contracts specifying profits based on particular transaction models in a way that may remove the incentive for private partners to adapt to new government systems. If the Defense Department wants to improve the efficiency of its business practices, it would do well to look to legislative or internal process reform before it looks to AI.
Another efficiency goal of the AI strategy is to “implement predictive maintenance and supply.” Predictive maintenance and supply creates efficiencies by predicting when equipment is likely to break and directing maintenance or parts to a problem just before it’s likely to occur. This works best when operating conditions are predictable enough to extrapolate usable data and required parts are a few hours away via express delivery. Unfortunately, when the user is in combat or 1,000 feet under the ocean, fixing a problem just before it is likely to occur may be too late. Under those circumstances, the consequences of a low-probability failure at the wrong time may be catastrophic. Of course, the Defense Department has many logistics needs that don’t involve extreme conditions of isolation or combat. But the Navy and the Air Force, in particular, already suffer from reduced readiness, at least partly because of previous efforts to find efficiencies by deferring “unneeded” maintenance and adopting just-in-time logistics. AI may or may not identify a better way to do these things — but will we believe the results produced by an algorithm if it says we need to spend more money supporting readiness? Or will we dismiss it the way we did when ship, squadron, and fleet commanders said the same thing?
It’s Not Just What You Buy: It’s How You Buy It
The AI strategy is more than rhetorical signaling to China. It is a nervous plea for private-sector collaboration without clear guidance about how the department plans to overcome sensitive and classified information barriers. While the Defense Department’s instinct to have these discussions behind SCIF doors is understandable at some level, the comforting security blanket of classification and “need to know” won’t cut it in discussions about AI. Unlike aviation and other 20th century technologies whose adaptation for war sped them to maturity, AI innovation is being led by civilian applications in the United States. The next generation of optical sensors and algorithms to support autonomous moral decision-making are as likely to emerge from the push for self-driving cars as from esoteric weapons. Certainly, discerning which private-sector algorithms to co-develop or fund should be a piecemeal, system-by-system consideration. But beyond this, the Department of Defense has to get better at identifying overlapping areas of interest and focusing resources on areas unique to defense.
If the Defense Department can identify the systems most suitable for “intelligentizing,” it could at least start working on the acquisitions processes needed to make that happen. Getting beyond secrecy also means finding new ways to either work in parallel with private industry, buy what is already available, or lead private industry down a path that is useful for the Defense Department. The military needs to identify, create, and voraciously commit to innovative ways of buying AI that may threaten existing cultural, bureaucratic, and contracting norms. The government simply does not have an internal workforce large or agile enough to identify which existing systems to shift, develop the intelligent tool for that system, implement it, maintain it, and then train its employees to operate it. All of this needs to happen with an eye toward touchy issues like supply chain security, data-sharing limitations, and privacy.
Negotiating public-private partnerships has already proven extremely controversial. The issues are both practical and cultural. Practically, the implementation of public-private partnerships is at the heart of the Joint Enterprise Defense Infrastructure contract lawsuits — the controversy over what company will win the bid for the Pentagon’s cloud computing solution. Working with the private sector means there will be winners and losers. In federal contracting, the losers tend to sue the government. This results in a stifling environment of risk-aversion and bureaucratic hedging. Culturally, many in the Silicon Valley workforce view the Defense Department with suspicion, as evidenced by Google’s decision to dissociate itself with Project MAVEN due to pressure over ethical concerns. Simply saying the Defense Department will be ethical — as the strategy does — is insufficient. It will take creative bravery by both corporate and Defense Department leaders to satisfy everyone’s concerns.
No One-Size-Fits-All Approach
The wide variety of tools and applications covered by AI will require diverse acquisition approaches. Sometimes the required acquisition processes will be department-wide; sometimes they will be service-specific. The size, ambition, and duration of these efforts will vary according to the culture of the service, the scope of the project, and the confidence of the leadership. Compare three examples, each driven by the culture, scope, and requirements of the service that developed them:
SOFWERX is Special Operation Command’s own small public maker shop. Its contracting and reach is specifically tailored to the command’s mission and culture of bespoke solutions to unique problems. Kessel Run, the Air Force’s software development program, draws upon the skills already present in the service’s workforce. It achieves agility and creativity by freeing workers from the institutional barriers associated with the Air Force’s larger footprint and bureaucratic service culture. The Defense Innovation Unit (DIUx) was one of a handful of experimental department-wide innovation programs intended to facilitate rapid contracting with the private sector. DIUx has now been renamed DIU — suggesting that it has been accepted as an institutionalized (rather than experimental) innovation vehicle.
The point is, no single approach will be sufficient. The AI strategy clearly advocates for a culture of experimentation and an environment of “fail-fast” ventures. To achieve this, the department will need risk-acceptant bureaucrats – which, frankly, sounds like an oxymoron. And yet, there are a few glimmers of hope.
The Navy has found a risk-acceptant leader in James “Hondo” Geurts, assistant secretary of the Navy for research, development, and acquisition. Geurts, prior to his appointment in late 2017, developed a reputation as a kind of innovation sherpa for start-up style rapid prototyping for Special Operations Command. His style is likely to ruffle feathers in a hulking platform-centric universe like the Navy’s fleet. Indeed, Geurts has already shaken up the bureaucracy with the announcement of the creation of the NavalX agility office, designed to connect innovators, leaders, and corporate partners to rapidly field new technologies.
Fast, Good, Cheap (Choose No More than Two)
As ambitious as it seems, the Defense Department’s AI strategy actually has elements that are quite conservative and risk-averse. Kudos to the authors for recognizing that failure is part of experimentation. But the strategy seems to assume that the likelihood and impact of failure can be mitigated by starting small and then scaling projects up. While starting small is wise, failure may nonetheless stem from the attempt to scale up in ways that small-scale implementations couldn’t foresee.
The focus on rapid implementation also creates an unacknowledged risk of invalid results: Experimentation needs time to account for omitted variables and differentiate between being lucky and being good. The strategy asserts that failures in implementing AI will occur quickly, early, and on a small scale – failures that are likely with any new technology. But by assuming that failure can somehow be limited to the early part of the process or to small-scale applications, the Defense Department’s strategy actually communicates a low tolerance for serious risk. What is the risk tolerance for implementing AI on a large scale in key mission areas?
A push to operationalize AI and intelligentize Defense Department operations requires many choices, all of which will involve high and low politics, ranging from great power competition to inter-service rivalry. They also will involve new questions of law and ethics. Fast answers to complex questions like these are rarely good answers, especially when value tradeoffs and risk tolerances aren’t made clear. Worse, the AI strategy, like the various service data strategies, is bureaucratically aligned within the office of the chief information officer. These executives are exactly the right people to answer tough questions of cyber security and information technology policy, but they may lack the institutional clout to persuade or prevail in broader, department-wide discussions about implementation of AI.
It’s possible that some of the specific choices and discussion of the appropriate level of risk are spelled out more clearly in the classified document. But the department is looking for public-private partnerships, and AI will profoundly change much more than just the U.S. military. As such, it’s important to start an unclassified public discussion about the trade-offs and risks rather than just pointing to its potential to “streamline,” “revolutionize,” or “accelerate” the business of national security.
The fact that the Defense Department now has a public AI strategy is good news. The fact that the strategy acknowledges the need for a culture of experimentation, including the likelihood of failure, is better news. But putting the department’s chief information officer, rather than the secretary of defense, in the lead, suggests that despite language about innovation, risk, and the consequences of losing the AI race, the Pentagon isn’t yet ready to commit to AI. There may be good reasons to be cautious regarding new technologies, but if we do so, we shouldn’t be surprised if we need to completely revamp our approach five or 10 years later. The H.M.S. Warrior, despite its ambition to be the world’s most powerful warship, reflected a mindset of caution and hedging about new technology. Will today’s efforts to integrate AI into the Department of Defense transform the department, or will we look back on them as just another screw-sloop?
Nina Kollars is associate professor of strategic and operational research at the Naval War College. She is also a founding member of the Cyber and & Innovation Policy Institute. Kollars’ current research investigates the security implications of the intersection of emerging technology, humans, and organizations. She is generally referred to as a bottom-up innovation scholar who looks at the role of practitioners in driving innovation.
Doyle Hodges is an associate professor at the U.S. Naval War College and a retired naval officer. His research focuses on civil-military relations, military professionalism, grand strategy, and the ethics of emerging technologies.
The views expressed here are the authors’ own and are not official positions of the Department of Defense, the Navy, or the Naval War College.
Image: U.S. Air Force Master Sgt. Barry Loo